Aarki is an AI Powered Mobile Marketing Platform
Mobile growth marketing is competitive. Consumer attention is valuable and so highly in demand. Aarki’s platform reduces the complexity by applying deep neural network models in concert with a fully managed service to help meet your marketing objectives.
Aarki’s 3 Pillars of Differentiation
Each pillar feeds off the other resulting in an ROI benefit that is greater than the sum of the parts
AI Platform
- Multi-level ML infrastructure with deep learning neural network.
- Dynamic Multi-object Bid Optimizer finds the best price for each bid maximizing ROI.
- Inventory quality control and fraud detection.
Privacy-first Engagement
- Embraced the no device ID world of targeting.
- 5M ad requests/sec from over 10B devices each with many contextual signals feeding an 800B row training model database.
- Creative hyper-targeting.
Unified Creative Framework
- An integrated creative + media agency working to discover and execute the most engaging ad concepts.
- Unified Creative Strategy seeks in-depth insights into outcomes based on ad attributes enabling optimized campaign decisioning.
AI Platform
Industry leading AI infrastructure for achieving optimal ROI at scale and with cost efficiency.
- Inventory Ad Request
- Step OneInventory Quality Control and Fraud Detection
We work hard to combat fraud so you can be confident in the quality of our results. This requires constant diligence and multiple layers of mitigation tactics.
Aarki inventory quality control and fraud detection ensures your campaign budget is not wasted by employing multiple layers of security:
- Internal algorithms constantly measure and evaluate user behaviors that are leading indicators of fraud or poor quality inventory.
- Fraudulent list of IPs and publishers updated with third-party data and consolidated data from all the MMPs.
- Step TwoPredict User Value with Deep Neural Network
The next stage in the process uses deep neural network (DNN) models to predict each impression or user value.
Aarki uses multi-stage DNN models because they ultimately yield better, more accurate prediction of the value of each impression. Each ML model learns features most important for that conversion event.
They identify installers and purchasers based on advertiser-publisher and advertiser-installed bundle (>50k) similarities obtained using app store APIs. The DNN navigates app similarity clusters in a high dimension, sparse space of possibilities. Only deep learning AI can do this!
- Step ThreeMulti-objective Bid Optimization
Once the user value has been determined for each bid request, Aarki employs multi-objective bid optimization algorithm to maximize the ROI on our winning bids. Most DSPs employ a bid shading algorithm that simply prices just under what they predict the winning bid is worth. Our algorithm is much more sophisticated as price is only one of the objectives we consider.
Over bidding is easy and done too often, underbidding results in underperforming and not effectively utilizing campaign budget - finding the right bid that will win, deliver on KPI goals, and pace at the correct rate to fully utilize campaign budget is hard!
The Aarki Multi-objective Bid Optimizer uses multiple dynamic variables such as campaign and inventory attributes, predicted user value and is segmented by CPM. All of these factors are used to guide multi-objective optimization around client KPIs - ROI or CPI. This enables us to dynamically acquire high value users with budget efficiency while also exploring untapped users and inventory outside the original model training scope.
- Optimal Winning Bid
We work hard to combat fraud so you can be confident in the quality of our results. This requires constant diligence and multiple layers of mitigation tactics.
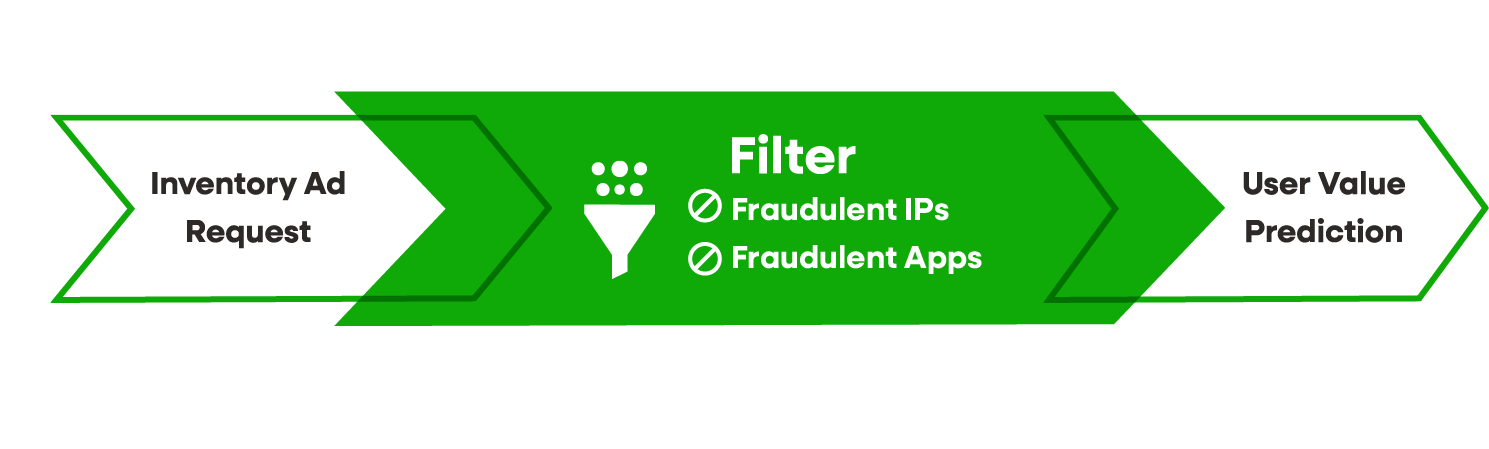
Aarki inventory quality control and fraud detection ensures your campaign budget is not wasted by employing multiple layers of security:
- Internal algorithms constantly measure and evaluate user behaviors that are leading indicators of fraud or poor quality inventory.
- Fraudulent list of IPs and publishers updated with third-party data and consolidated data from all the MMPs.
The next stage in the process uses deep neural network (DNN) models to predict each impression or user value.
Aarki uses multi-stage DNN models because they ultimately yield better, more accurate prediction of the value of each impression. Each ML model learns features most important for that conversion event.
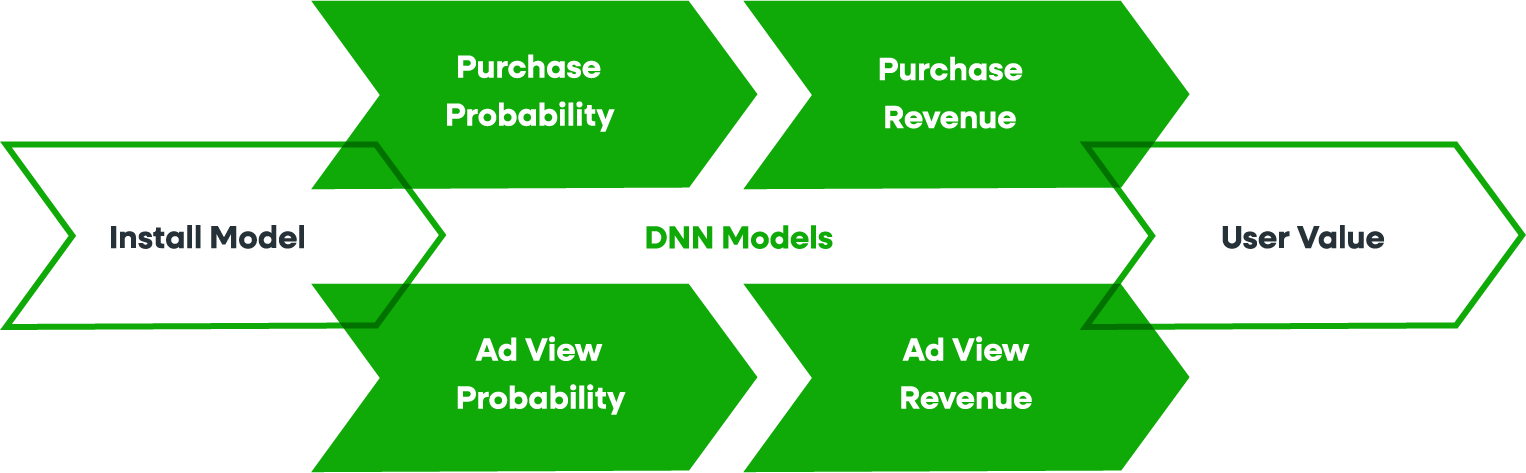
They identify installers and purchasers based on advertiser-publisher and advertiser-installed bundle (>50k) similarities obtained using app store APIs. The DNN navigates app similarity clusters in a high dimension, sparse space of possibilities. Only deep learning AI can do this!
Once the user value has been determined for each bid request, Aarki employs multi-objective bid optimization algorithm to maximize the ROI on our winning bids. Most DSPs employ a bid shading algorithm that simply prices just under what they predict the winning bid is worth. Our algorithm is much more sophisticated as price is only one of the objectives we consider.
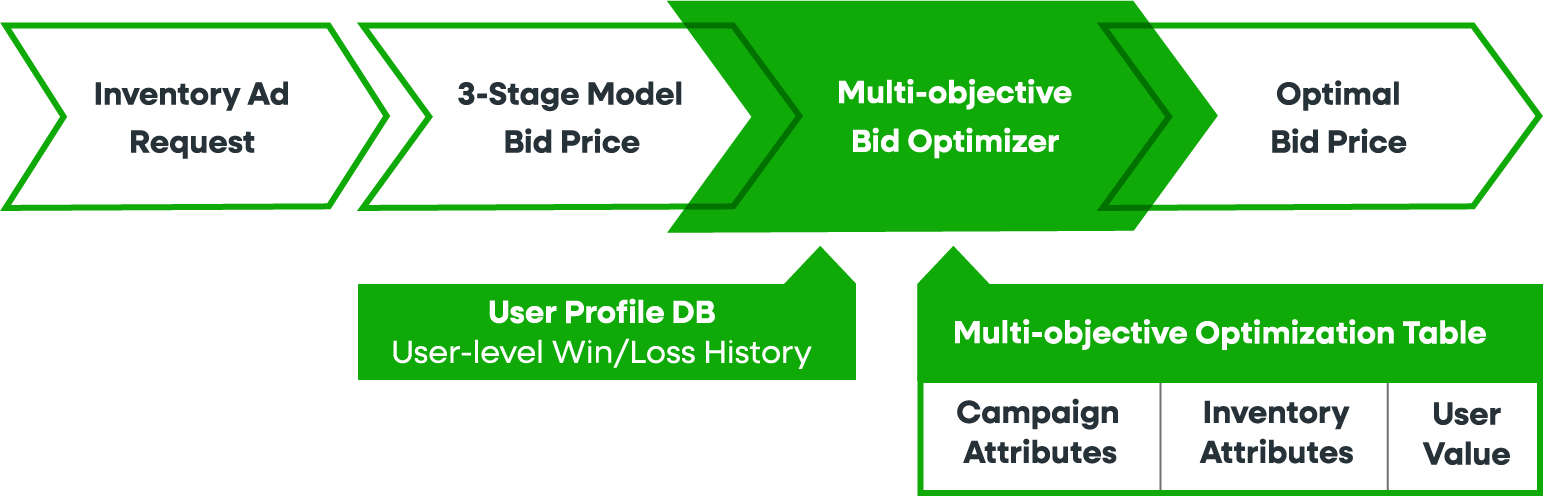
Over bidding is easy and done too often, underbidding results in underperforming and not effectively utilizing campaign budget - finding the right bid that will win, deliver on KPI goals, and pace at the correct rate to fully utilize campaign budget is hard!
The Aarki Multi-objective Bid Optimizer uses multiple dynamic variables such as campaign and inventory attributes, predicted user value and is segmented by CPM. All of these factors are used to guide multi-objective optimization around client KPIs - ROI or CPI. This enables us to dynamically acquire high value users with budget efficiency while also exploring untapped users and inventory outside the original model training scope.
Privacy-first Engagement
User
Acquisition
Aarki’s second pillar of differentiation is our solutions for privacy-first strategic cohorting for user acquisition campaigns. We are ID-less today - SKAN 4 compliant. We leverage trillions of contextual signals and use ML to derive correlations with historical outcome data. This is the foundation for creating highly detailed, dynamic behavioral cohorts.
For each cohort, we use ML and collaborate closely with our creative team to devise the most optimal creative strategy, a methodology we refer to as ML-driven Creative Targeting™.
We have access to 5M+ ad requests per second from 10B mobile devices globally each with contextual data such as: device type, OS, app, genre, time of day, region, and many more.
Aarki DB of historical contextual signals correlated with behavioral outcome data.
We create dynamic behavioral cohorts to enable efficient optimizations, scale, and ML-driven Creative Targeting.
Re-Engagement
For re-engagement campaigns we do retargeting with our clients first-party segments extended with our data. We can use any number of user or audience-based strategies.
User Segmentation
- Geographic
- Demographic
- Psychographic
- Behavioral
Audience Segmentation
- Time Spent
- Value
- Behavior
- Location
Unified Creative Strategy™
Creative is core to our platform, we’ve established a Unified Creative Strategy, a pillar that encompasses:
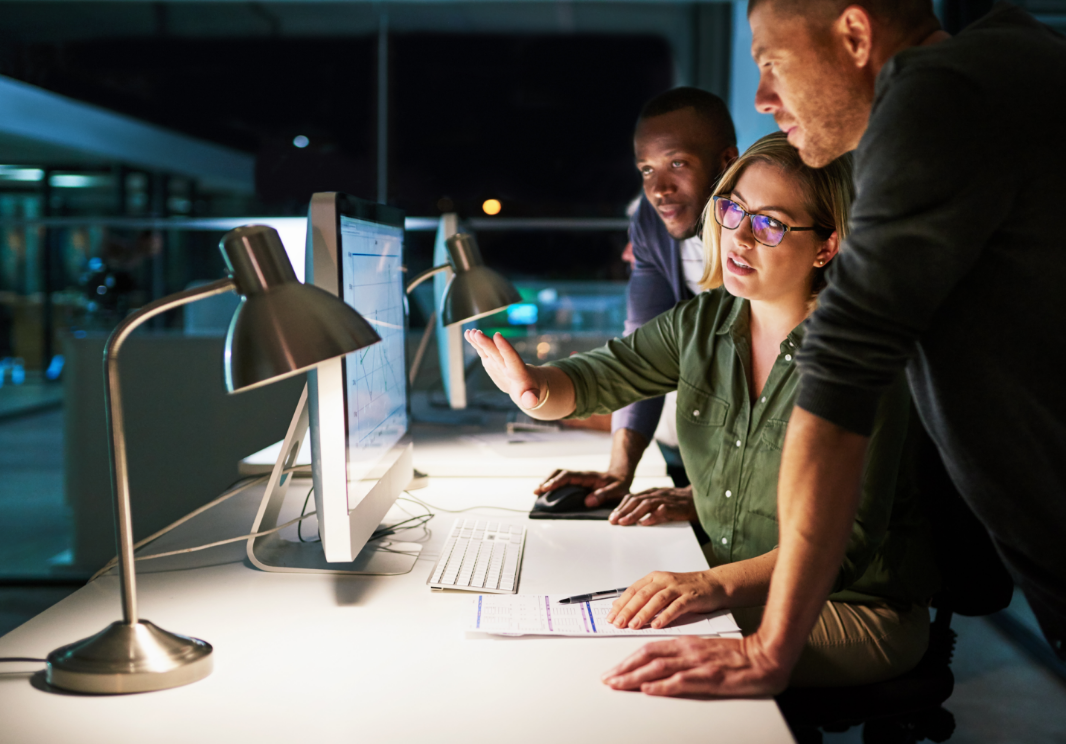
High domain IQ creative team delivers creative and strategic solutions. Can benchmark tactics and results across regions and categories.
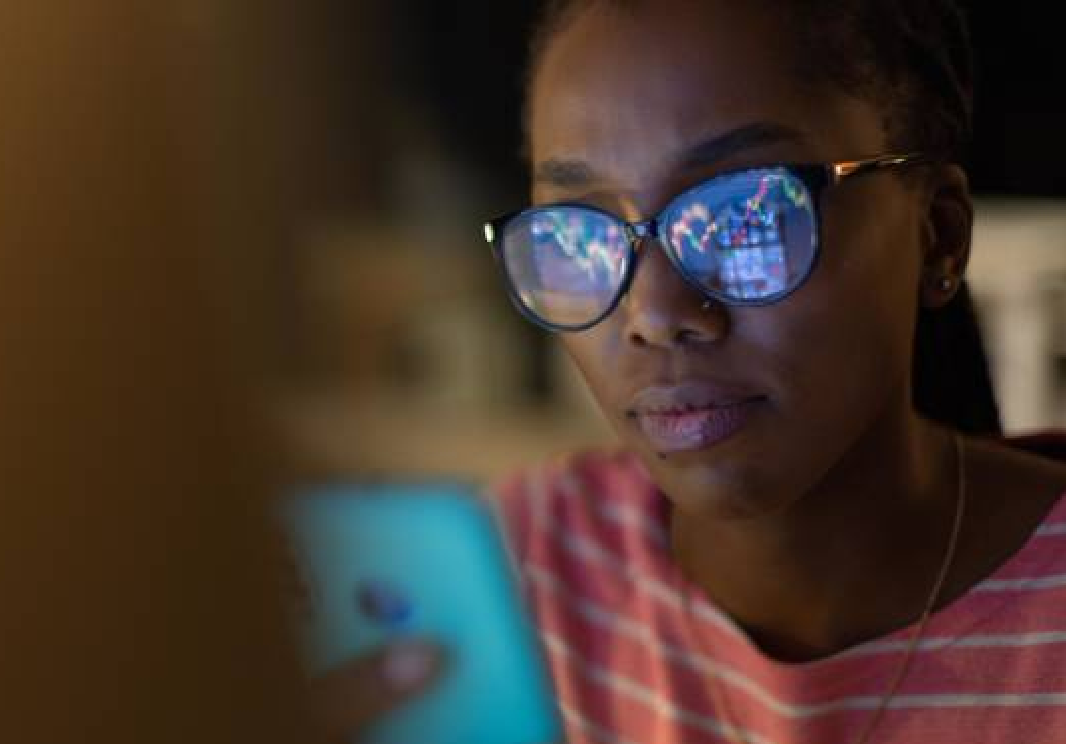
Decompose each creative into measurable dimensions of effectiveness based on performance outcomes - share those best performing dimensions across all campaign line items to derive new and better ad creatives.
Integrating creativity and technology as a white glove client service for enhanced advertising performance
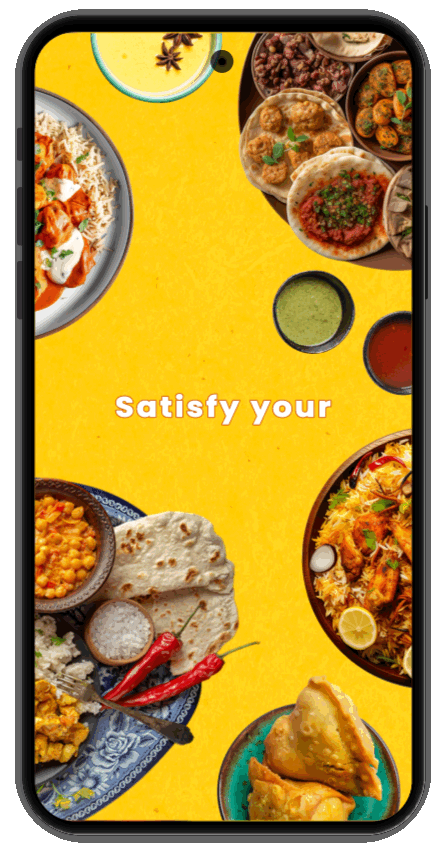
Video
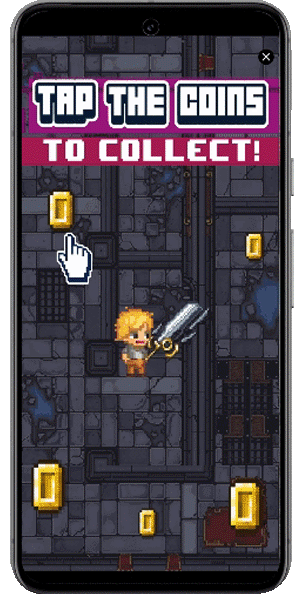
Playable
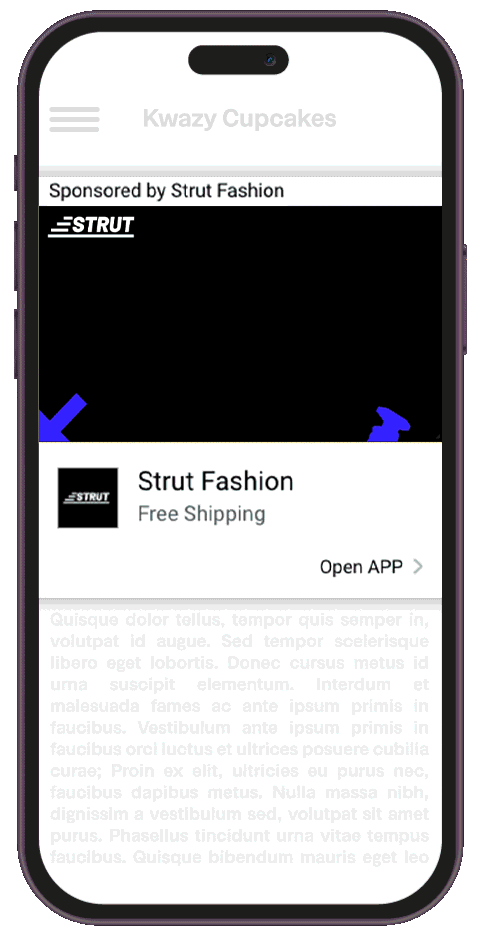
Native
The Agency
The agency team also utilizes a variety of creative optimization tactics to Improve install rates and ROI
Weight Allocation
Performance-based budget allocation based on theme and mechanics.
Creative Selection Algorithm
Automatically tests and allocates creative weights for best performing creatives.
Variant Testing
Extends the life of a creative by adding small changes to existing concepts.